As of March 9, 2022, the severe acute respiratory syndrome coronavirus 2 (SARS-CoV-2), the causative agent for the coronavirus disease 2019 (COVID-19) pandemic, has infected over 446 million people and caused over 6 million deaths worldwide, with an estimated mortality rate of 1.5%.
Study: A predictive model for hospitalization and survival to COVID-19 in a retrospective population-based study. Image Credit: Cryptographer / Shutterstock.com
Background
COVID-19 vaccines have proven effective in reducing hospitalization and mortality rates. Periods of extensive SARS-CoV-2 transmission during the ongoing pandemic, which have also been referred to as ‘waves,’ have put a strain on hospital resources. This strain has been largely due to the unprecedented number of COVID-19 cases requiring critical care, which exceeded the intensive care unit (ICU) capacity.
During these peaks of SARS-CoV-2 transmission, mortality rates were particularly heightened as a result of the overburdened health care systems. Thus, going forward, prompt risk stratification, planned clinical management, and optimized use of resources are crucial in the management of the COVID-19 pandemic.
Electronic medical records (EHR) serve as comprehensive guides for the precise triage and coherent management of COVID-19 patients. Artificial intelligence has been used to predict the prognosis of SARS-CoV-2 infected patients utilizing health and demographic data collected from healthcare systems.
However, in most instances, these data are biased, as the proportion of patients with severe disease episodes remains small. As a result, supervised machine learning may not be a balanced model to predict COVID-19 outcomes.
About the study
A recent study published on the medRxiv* preprint server presents a new technique to solve unbalanced problems for the efficient management of SARS-CoV-2 infected patients according to comorbidities, age, and sex based on data available from the Regional Health System in Spain.
Moreover, this technique involved the use of machine learning to develop models to determine whether a newly-diagnosed COVID-19 patient would require hospitalization and predict their prognosis.
As the data were highly imbalanced due to the fewer number of expired and hospitalized patients as compared to discharged and outpatients, a new ensemble-based and imbalance-aware machine learning method termed Identical Partitions for Imbalance Problems (IPIP) was proposed. For each question of interest, two IPIP models were created and evaluated with five-fold cross-validation.
Classifying COVID-19 patient subtypes
The present study was conducted between January 4, 2020, to February 4, 2021, and included patients diagnosed with COVID-19, as confirmed by a positive antigen test or reverse-transcriptase polymerase chain reaction (RT-PCR) assay from pharyngeal or nasal swab specimens.
An exploratory analysis of 86,867 SARS-CoV-2 positive patients showed that 93.7% were outpatients, with 5.4% hospitalized in the non-ICU, while 0.85% were ICU patients. The most common symptoms included cough in 49.9% of the cases, headache in 38.3%, and myalgia in 36%.
The participants were classified into three types. The outpatient prototype was a female aged 38 years with two affected systems and two chronic pathologies, with common comorbidities including arterial hypertension, obesity, asthma, and depression. Comparatively, the typical hospitalized non-ICU patient was a male aged 62 years with four affected systems and five chronic pathologies, with more frequent comorbidities.
The prototype ICU patient was a 62-year-old male with three affected systems and five chronic pathologies, with the most frequent comorbidities including arterial hypertension, diabetes mellitus, obesity, and osteoarthritis. ICU patients had double the mortality rate as compared to hospitalized non-ICU patients.
Further investigations were carried out to differentiate survivors from the deceased. The prototype survivor was a female aged 39 years with two affected systems and two chronic pathologies and comorbidities similar to the outpatients.
Comparatively, the deceased prototype was a male aged 83 years with five affected systems and eight chronic pathologies, whose most frequent pathology was arterial hypertension (75.64%). Additional comorbidities of this patient type included diabetes mellitus, depression, osteoarthritis, and obesity.
Three variables including age, comorbidity, and affected systems were relevant to the final status of a patient. Increasing age, the number of comorbidities, and affected organ systems heightened the probability of death. A similar relationship could be deduced between outpatients, ICU patients, and hospitalized non-ICU patients.
Males were found to have a higher mortality rate than females. Higher risk comorbidities included renal insufficiency, heart failure, stroke, dementia, and ischemic cardiomyopathy. COVID-19-related deaths could not be correlated to the presence of asthma, osteoporosis, or osteoarthritis.
The accuracy of machine-learning models
Multiple machine-learning models were generated to predict patients’ need for hospitalization and final condition. To handle the unbalanced data, two machine learning algorithms including Logistic Regression and Random Forest were assessed with or without considering IPIP.
The model using Logistic regression with IPIP (LR-IPIP) provided the best result for predicting the final condition of a patient with a balanced accuracy. The result showed that the ROC-AUC for the unbalanced dataset predicted by this model was 0.937.
The most important determinants of the final condition of patients using the RL-IPIP model included age, sex obesity, osteoarthritis, and the number of affected systems.
A training dataset was used to develop a model for assessing the hospitalization need and a test dataset was used for its evaluation. LR-IPIP model gave the best results.
Using the RL-IPIP model, the hospitalization need was predicted with a balanced accuracy of 0.72 for the balanced dataset and between 0.71-0.73 for imbalanced datasets. ROC-AUC for the unbalanced dataset predicted by this model was 0.746. Age, sex, renal insufficiency, depression, and the number of chronic diseases were relevant characteristics obtained by the RL-IPIP model.
Feature importance for the final models. NSA is the number of systems affected and NCD is the number of chronic diseases.
Conclusions
The current study developed and analyzed machine-learning-based models that could predict the final state of SARS-CoV-2 infected patients with high accuracy, as well as assess patients’ need for hospitalization with reasonable precision. Moreover, the class imbalance was solved by developing a new algorithm known as IPIP.
The proposed LR-IPIP model could be used to efficiently manage COVID-19 patients who have limited access to healthcare resources. The predictive models, together with corresponding web applications, are accessible at GitHub for future use in future COVID-19 waves or other viral respiratory diseases.
*Important notice
medRxiv publishes preliminary scientific reports that are not peer-reviewed and, therefore, should not be regarded as conclusive, guide clinical practice/health-related behavior, or treated as established information.
- Cisterna-García, A., Guillén-Teruel, A., Caracena, M., et al. (2022). A predictive model for hospitalization and survival to COVID-19 in a retrospective population-based study. medRxiv. doi:10.1101/2022.03.02.22271552. https://www.medrxiv.org/content/10.1101/2022.03.02.22271552v1.
Posted in: Device / Technology News | Medical Science News | Medical Research News | Disease/Infection News | Healthcare News
Tags: Antigen, Artificial Intelligence, Assay, Asthma, Cardiomyopathy, Chronic, Coronavirus, Coronavirus Disease COVID-19, Cough, Critical Care, Dementia, Depression, Diabetes, Diabetes Mellitus, Electronic Medical Records, Headache, Health Care, Healthcare, Heart, Heart Failure, Hospital, Intensive Care, Machine Learning, Mortality, Obesity, Osteoarthritis, Osteoporosis, Pandemic, Pathology, Polymerase, Polymerase Chain Reaction, Respiratory, SARS, SARS-CoV-2, Severe Acute Respiratory, Severe Acute Respiratory Syndrome, Stroke, Syndrome, Triage
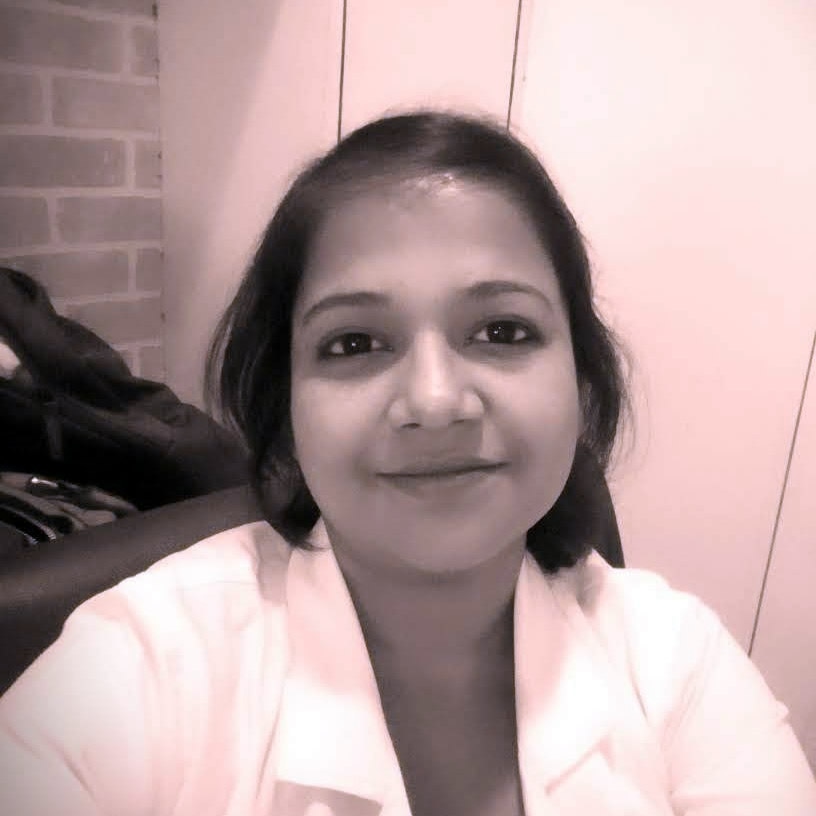
Written by
Nidhi Saha
I am a medical content writer and editor. My interests lie in public health awareness and medical communication. I have worked as a clinical dentist and as a consultant research writer in an Indian medical publishing house. It is my constant endeavor is to update knowledge on newer treatment modalities relating to various medical fields. I have also aided in proofreading and publication of manuscripts in accredited medical journals. I like to sketch, read and listen to music in my leisure time.
Source: Read Full Article